Fitting N-mixture models to count data with unmodeled heterogeneity: bias, diagnostics, and alternative approaches
Abstract/Summary
Monitoring animal populations is central to wildlife and fisheries management, and the use of N-mixture models toward these efforts has markedly increased in recent years. Nevertheless, relatively little work has evaluated estimator performance when basic assumptions are violated. Moreover, diagnostics to identify when bias in parameter estimates from N-mixture models is likely is largely unexplored. We simulate count data sets using 837 combinations of detection probability, number of sample units, number of survey occasions, and type and extent of heterogeneity in abundance or detectability. We fit Poisson N-mixture models to these data, quantified the bias associated with each combination, and evaluated if the parametric bootstrap goodness-of-fit (GOF) test can be used to indicate bias in parameter estimates. We also explore if assumption violations can be diagnosed prior to fitting N-mixture models. In doing so, we propose a new model diagnostic, which we term the quasi-coefficient of variation (QCV). N-mixture models perform well when assumptions are met and detection probabilities are moderate (i.e., ≥0.3), and the performance of the estimator improved with increasing survey occasions or sample units. However, the magnitude of bias in estimated mean abundance with even slight amounts of unmodeled heterogeneity was substantial. The parametric bootstrap GOF test did not perform well as a diagnostic for bias in parameter estimates when detectability and sample sizes were low. The results indicate the QCV is useful to diagnose potential bias and that potential bias associated with unidirectional trends in abundance or detectability can be diagnosed using Poisson regression. This study represents the most thorough assessment to date of assumption violations and diagnostics when fitting N-mixture models using the most commonly implemented error distribution. Unbiased estimates of population state variables are needed to properly inform management decision making. Therefore, we also discuss alternative approaches to yield unbiased estimates of population state variables using similar data types, and we stress that there is no substitute for an effective sample design that is grounded upon well-defined management objectives.
Publication details
Published Date: | 2018-02 |
Outlet/Publisher: | Ecological Modelling 10.1016/j.ecolmodel.2018.02.007 |
Media Format: |
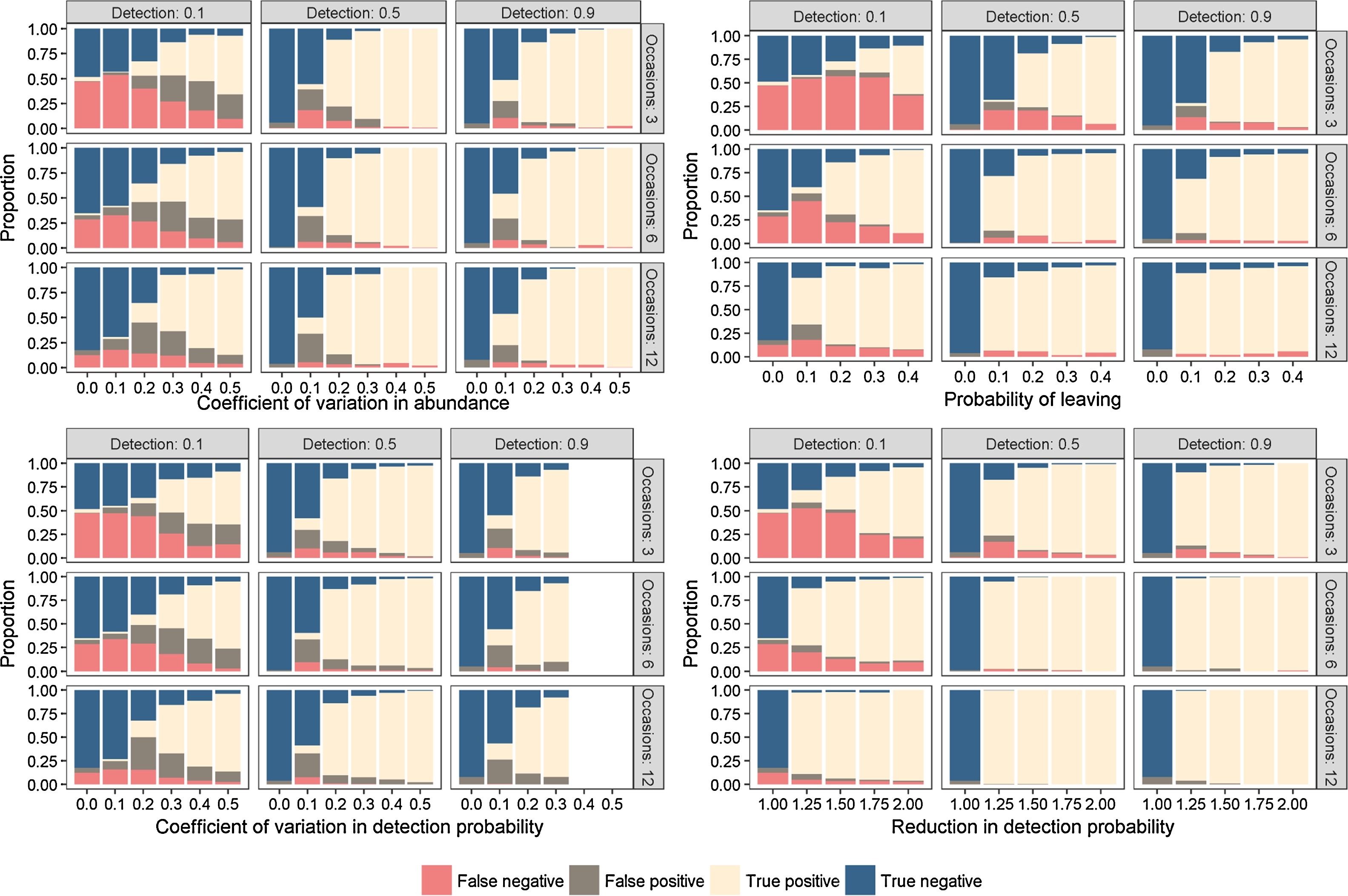
Proportion of iterations that were true positives, true negatives, false positives, and false negatives when evaluating if a parametric bootstrap goodness-of-fit test can diagnose bias in parameter estimates from N-mixture models for scenario 2 (top left), scenario 3 (top right), scenario 4 (bottom left), and scenario 5 (bottom right).
Photo by: Adam Duarte